7.1 Research Terminology
Nicholas Walliman, in his handbook Research Methods: The Basics, defines research methods as “the tools and techniques for doing research” (2011). These techniques include collecting, sorting, and analyzing the information and data you find. The better the tools and more comprehensive the techniques you employ, the more effective your research will be. By extension, the more effective your research is, the more credible and persuasive your argument will be.
Here are some basic terms and definitions you should be familiar with:
Research: the systematic process of finding out more about something than you already know, ideally so that you can prove a hypothesis, produce new knowledge and understanding, and make evidence-based decisions
Research Methods: techniques of collecting, sorting, and analyzing information/data
Data: bits of information.
The typical kinds of research sources you will use can be grouped into three broad categories:
- Primary Sources: research you might conduct yourself through surveys, observations, interviews, etc. These can also include published statistical data, historical records, legal documents, firsthand historical accounts, and original creative works.
- Secondary Sources: written sources that discuss, analyze, and interpret primary data, such as published research and studies, reviews of these studies, meta-analyses, and formal critiques.
- Tertiary Sources: reference sources such as dictionaries, encyclopedias, and handbooks that provide a consolidation of primary and secondary information. They are useful to gain a general understanding of your topic and major concepts, lines of inquiry, or schools of thought in the field.
Data can be categorized in several ways:
Primary data Data that have been directly observed, experienced and recorded close to the event. These are data that you might gather yourself by
Note: Primary research done in an academic setting that includes gathering information from human subjects requires strict protocols and will likely require ethics approval. Ask your instructor for guidance and see Chapter 5.4 Human Research Ethics. |
Secondary Data Comes from sources that record, analyze, and interpret primary data. It is critical to evaluate the credibility of these sources. You might find such data in
|
Quantitative Data Use numbers to describe information that can be measured quantitatively. These data are used to measure, make comparisons, examine relationships, test hypotheses, explain, predict, or even control. |
Qualitative Data Use words to record and describe the data collected; often describe people’s feelings, judgments, emotions, customs, and beliefs that can only be expressed in descriptive words, not in numbers. These include “anecdotal data” or personal experiences. |
Research methods are often categorized as quantitative, qualitative, or “mixed methods.” Some projects, like a feasibility project, require the use of a more scientific method of inquiry, observation, quantitative data collection, analysis, and conclusions to test a hypothesis. Other kinds of projects take a more deductive approach and gather both quantitative and qualitative evidence to support a position or recommendation. The research methods you choose will be determined by the goals and scope of your project, and by your intended audience’s expectations.
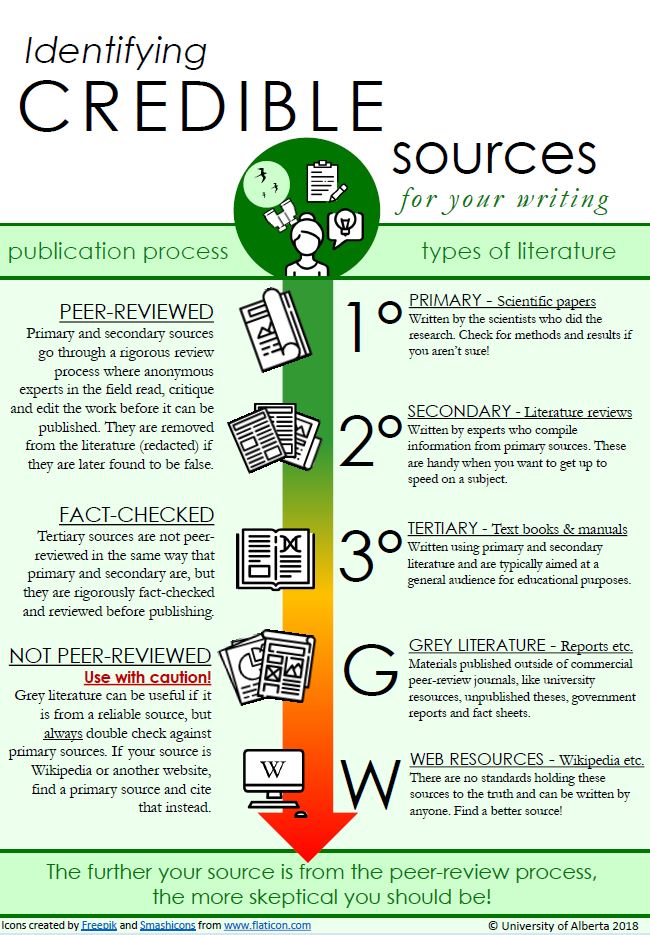
Exercise 7.1.1
More specific methodologies, such as ways to structure the analysis of your data, include the following:
- Cost/benefit Analysis: determines how much something will cost vs what measurable benefits it will create.
- Life-cycle Analysis: determines overall sustainability of a product or process, from manufacturing, through lifetime use, to disposal. (You can also perform comparative life-cycle analyses, or specific life cycle stage analysis.)
- Comparative Analysis: compares two or more options to determine which is the “best” solution (given specific problem criteria such as goals, objectives, and constraints).
- Process Analysis: studies each aspect of a process to determine if all parts and steps work efficiently together to create the desired outcome.
- Sustainability Analysis: uses concepts such as the “triple bottom line” or “three pillars of sustainability” to analyze whether a product or process is environmentally, economically, and socially sustainable.
In all cases, the way you collect, analyze, and use data must be ethical and consistent with professional standards of honesty and integrity. Lapses in integrity can not only lead to poor quality reports in an academic context (poor grades and academic dishonesty penalties), but in the workplace, these lapses can also lead to lawsuits, loss of job, and even criminal charges. Some examples of these lapses include
- fabricating your own data (making it up to suit your purpose),
- ignoring data that disproves or contradicts your ideas,
- misrepresenting someone else’s data or ideas, and/or
- using data or ideas from another source without acknowledgment or citation of the source.
(Plagiarism and You, 2020)
Failing to cite quoted, paraphrased or summarized sources properly is one of the most common lapses in academic integrity, which is why your college writing subjects, COM101 and COM111, spent considerable time and effort to give you a sophisticated understanding of how and why to avoid plagiarizing, as well as the consequences of doing so. If you would like to review this information, see Chapter 8.2: Integrating Source Evidence into Your Writing, and consult Seneca College’s Policy on Academic Integrity and Seneca’s Student Resources on Academic Integrity.
Exercise 7.1.2
References
Algonquin College Library. (2020). Plagiarism and you [Video]. Youtube. https://www.youtube.com/watch?v=eYhGPHAnFak&t=34s
Nylund, C. (2018). [Image]. Identifying credible sources. Open Education Alberta, https://openeducationalberta.ca/libraryskills200/chapter/evaluating-resources/
Seneca College. (n.d.) Academic Integrity Policy. https://www.senecacollege.ca/about/policies/academic-integrity-policy.html
Seneca College. (n.d.). Student Resources. Academic Integrity at Seneca College. http://open2.senecac.on.ca/sites/academic-integrity/for-students/
Walliman, N. (2011). Research methods: The basics. New York: Routledge.