2.3 Critical Issues in GenAI
GenAI Considerations
While the generative pretrained transformer (GPT) upon which the ChatGPT model is based is not new, OpenAI’s launch of ChatGPT in November 2022 marked the fastest recorded adoption of a technology tool to date. In October 2023, the site had 1.7 billion visits in one month, marking the highest level of usage of any application (Carr, 2023). The rapid proliferation of tools and advancements in the technology saw over 100 leaders in AI technology write an open letter urging a collective pause on AI developments more powerful than GPT 4 to give time for security and safety features to develop and for the creation of regulations and governance structures. The need for such regulation or governance extends to full nations, organizations as well as science and education.
The innovation and creativity in the area of genAI are exciting. These applications can assist with ideation and writing, video and presentation production, research on small and large scales, data analysis and interpretation, image generation, music production and more. ChatGPT can also interact with humans verbally and via camera in such as way as to mimic natural human interactions. However, these systems do not come without limitations or ethical challenges. Some of these challenges speak to the specifics of academic contexts – like academic integrity – while others intersect with communities, organizations, governments, the environment, and humanity as a whole. Broader issues related to genAI include privacy of personal data, risks of misinformation, existential risks, concerns about job dislocation or loss, environmental costs, labour exploitation, and copyright. And specific to the technology, many AI experts have documented alarming concerns relating to the size and scale of large language models, misinformation, AI misalignment, and existential risks to humanity.
Whereas we may, as typical users, have little if any control over how the models are trained, what data is used for training, and how the algorithms process the data, we nonetheless have some control over how we use the models, their applications, and their output. As the humans in the process, we have the responsibility to maintain agency in the human-AI interaction, and we have the obligation to engage in the process by applying due diligence, ethical standards, and a critical perspective. And the way to embrace this human agency is to develop literacy about genAI and apply sound principles for use.
The ethical issues surrounding the development, operation, use, and maintenance of genAI models and applications are numerous and complex. This chapter offers an overview of key areas of concern; for more detailed discussions of the topics, please refer to the References, which provides additional resources for consideration.
Bias
GenAI tools are trained on a range of data, and some frontier models like GPT and other large language models (LLMs) were trained on a wide range of sources including internet sites, books, reports, and datasets. Biases inherent in the training data — those that may discriminate against or marginalize underrepresented, minority, and equity-deserving groups — may appear in the outputs generated by these tools. While efforts have been made by companies like OpenAI to create ‘guardrails’ to prevent hateful and discriminatory results from being generated, the risk of bias persists in the limitations of the training data itself. That is to say existing biases in the training data may make a discriminatory result statistically more likely, so the genAI application is more likely to produce that result.
For instance, in a prompt to generate a story about slaying a dragon, the probabilistic result is to have a prince (and not a princess) slay the dragon because that is the most common pattern in the training data. This somewhat innocuous example points to the broader risk of unexamined bias in the responses; that is to say, the result doesn’t have to be hate speech to be harmful, nor does it have to be extreme to be biased. Take a look at this video from the London Interdisciplinary School (2023) for more insight.
Users, either consciously or unconsciously, may also inject bias into their prompts, resulting in biased output. Being aware of one’s own attitudes, beliefs, and prejudices will enable the user to reflect on the situation and mitigate any potential harm through a thorough review of both the prompt and output.
As communicators, we need to be thoughtful about the ways these biases might be perpetuated or left unexplored when we use genAI output in all types of documents and media.
Take Action: Completing a bias check for all prompts and output, and editing the content to remove bias is an important obligation for all users of genAI. To do this, you must be well informed about the issues relating to bias, so taking the steps to become literate on issues relating to representations of race, socioeconomic status, gender, ethnicity, ability, and other forms of bias will help you develop a keen awareness of what to watch for in output.
Hallucinations
LLMs make things up, and they convey those falsehoods with such a confident tone that humans can be fooled into accepting the fake content. As probabilistic models, they are designed to generate the most likely response to any given prompt. Given that these tools do not ‘know’ anything, and are — in most instances — limited in their ability to fact check, the responses generated can include factual errors and invented citations/references. This known phenomenon has been termed ‘hallucination,’ and is one persuasive reason to evaluate and fact-check all output an LLM produces. Take a look at this video below to find out about why hallucinations occurs.
In a recent Forbes article, Michael Ringman (2023) describes some measures being taken by developers to mitigate or prevent these hallucinations. These measures include setting up guardrails to create topical, safety, and security constraints for the models. He also suggests that monitoring and fine tuning them to produce more accurate results is essential. However, ultimately we as users are responsible for whatever output we use in our documents. Applying our own knowledge and skills in the critical evaluation of the output is key to ensuring that whatever output we transfer to our documents is factual and otherwise accurate. As communicators, we must become active agents in the process of ensuring accuracy.
Take Action: Apply a healthy skepticism when reviewing LLM output and adopt a consistent practice of checking outputs against verified sources. Verify outputs for claims lacking evidence and/or taken out of the context of the original argument. Then follow up to correct and/or support claims ensuring that they align with the original intent.
MisInformation, Disinformation, and Malinformation (MDM)
The ability of genAI to create realistic and plausible text, video, audio, and code makes the creation of false, biased, or politically motivated media faster and easier to produce. For example, the technology currently enables bad actors to realistically represent people speaking words that they did not speak and can place them in visual contexts (such as in photos or videos) where they have never been. When put out into the world, deepfakes and other such media can have profound impacts on decision making, social and political attitudes and movements, political races and elections, economies, journalistic integrity, and emotional states, to name a few areas.
So what are misinformation, disinformation, and malinformation (MDM)?
Misinformation: Misinformation is the use of false information in credible formats and contexts, which leads consumers to believe that it is true. Such information is typically not intended to cause harm (Government of Canada, 2022).
Disinformation: Disinformation is the deliberate use of false information to deceive consumers. Such information may lead people, organizations, and governments to make decisions that are based on manipulation and errant guidance. (Government of Canada, 2022)
Malinformation: Malinformation is often fact-based information presented in ways that deliberately mislead consumers. (Government of Canada, 2022)
MDMs have become significant influencers in today’s information economy. This video summarizes several key concerns.
The Government of Canada’s “How to identify misinformation, disinformation, and malinformation” (2022) offers some great information about MDM along with suggestions on how organizations and consumers can take action to manage it. Sohaib et al. (2023) suggest practical steps for addressing the challenges posed by deepfakes. They range from technical detection and authentication to collaborative filtering approaches to end user vigilance. Here are some of their suggestions on what you can do as a typical user within a workplace context.
Take Action:
- Develop routine vigilance, skepticism, and critical thinking using a keen eye for finding fake information. People speaking words in videos that normally they would never be caught saying or noticing flawed images, such as of people with seven fingers on one hand for example or other visual inconsistencies for videos and images, are good indicators that what you are viewing may constitute MDM.
- Become AI-literate on the issues (such as discussed in this chapter). Once you know what to look for you can then be proactive in mitigating the impact of misrepresentations on you and your decision making.
- Check the facts. If they don’t match up with verifiable sources, then don’t make use of the material.
- In community-driven contexts such as social media or other collaborative platforms or message boards, Sohaib et al. (2023) suggest that you establish moderation so as to filter out potential MDM. In addition, they suggest implementing crowd-sourcing (when a large group of people are marshalled to work on one task) to verify facts when the context warrants and makes possible such an approach. Real time fact checking for live events is another way that MDM can be combatted.
Knowledge Check
Copyright
As discussed previously, genAI models have been trained on a wide range of data from many disciplines and many cultures and areas of life. Many of these models include in their datasets content created and shared publicly – such as internet sites and social media like Twitter or Reddit – as well as that created by artists or users. Whereas much of the output created by the models does not contain language or images that can be linked directly to copyrighted work (which raises the important issue of tracing provenance or origin), some 8000 creators are insisting that they be compensated fairly if genAI models are to be trained on their work (Associated Press, 2023). Ongoing lawsuits related to copyright filed by artists are challenging the inclusion of creative works in these training datasets, while others are navigating questions around what might be fair use.
Efforts are being made to address the copyright issue relating to training data. For example, organizations and models can be certified by Fairly Trained for exclusively making use of fairly obtained data. The KL3M, created by 273 Ventures, is the first certified LLM that has been created with clean, non-toxic intellectual property, which KL3M (2024) characterizes as content that has a clear provenance and is free of copyright issues, synthetic (made up) data, and toxic sources. While its performance has yet to match that of GPT class models, it is having a promising start.
For more information on the topic of copyright and genAI along with best practices, please see Seneca’s Copyright and Generative AI. The following are additional steps you can take.
Take Action:
- Ensure that the genAI output you use contains accurately cited information. For example, if you see a statement that claims that productivity has increased by 40% with the use of LLMs in office tasks, you would want to then research that statistic to find out its origin. Which study did that data first appear in? Who are the authors of that study? Is that bit of data accurately represented in the output? You would then correct the statement (if necessary) then apply citation practices to reference that data.
- When choosing platforms to work with, when possible, choose one that prioritizes the creators of original work. Some platforms, like Adobe Firefly, are participating in initiatives that aim to protect and reward contributors, while others are tailoring their datasets to include content with consent for inclusion explicitly obtained.
- Choose to work within protected environments. While some people and organizations will refuse to use genAI due to the uncertainty relating to possible litigation for use of copyrighted content (if ever provenance becomes traceable), others will choose to work within protected AI environments. Microsoft, for example, has established a Copyright Commitment for Azure OpenAI customers, which protects them from copyright litigation and its costs (Smith, 2023). Choosing to work within this framework gives no rest for those concerned about the moral implications of using LLM output and those wanting free and open access to a variety of genAI applications, but from a business standpoint, it gives some reassurance to organizations and end users as they adapt to the unavoidable ubiquitous presence of the technology.
Environmental Concerns
While AI can be used to help find solutions to mitigate climate change (Cho, 2023), the exact environmental costs of genAI models are difficult to know but they have been widely acknowledged to be exorbitant. Data centers where models are trained and run need considerable energy and amounts of water to cool processors and generate electricity (Crawford, 2024). Long term, the impact on the environment could be ruinous.
At a July 2023 event, Sam Altman, Chief Executive Officer of OpenAI, stated that the cost of training ChatGPT cost over $100 million, and the cost is increasing along with the model’s size and capabilities (Smith, September 2023). The size of the model, the training approach used, and the capabilities of the tool influence how much energy the model uses (Cho, 2023; Smith, 2023). Likewise, energy needs exist for training a model and for using it.
Some prominent companies – like Google and Microsoft – have also pledged to be carbon neutral or carbon negative in a way that – ostensibly – would account for the energy use of their genAI applications Gemini and Copilot, respectively. That said, the known energy-consumption and overall environmental impact of these tools should not be a limitation left unexamined. See what Sam Meredith, Correspondent of CNBC International, has to say on the topic in this video.
Developers are aware of the enormous environmental cost involved in sustaining operations, so with each iteration of the models, they are making efforts to reduce the costs. Peter Gostev, Head of AI at Moonpig, depicts in a visualization the differences in pricing representing an 80% in savings in one year with the evolution from ChatGPT-3.5 to 4o: OpenAI Models – Elo & Pricing Over Time | Flourish.
As a community at Seneca, we have an opportunity to make a difference by contributing to carbon offsetting programs and to educating ourselves on the environmental cost of these tools. As professionals who will be contributing to an organization’s sustainability practices, you too have a role to play. Below are some initiatives relevant to end users suggested by Professors Kumar and Davenport in their 2023 Harvard Business Review article.
Take Action:
- Be discerning on when you use a genAI application. Is it worth using an expensive machine to create content you can easily create yourself? Using it for tasks that will augment not replace your abilities is perhaps one way to decide when and when not to use it. Or use it for tasks, like analyzing a large dataset, that you simply could not do within a reasonable amount of time.
- Encourage your organization to use existing large language models and not to create their own. Using existing ones saves the planet from the significant environmental, material, and financial costs involved in creating a new one.
- When an existing model does not exactly suit your organization’s needs, ask your organization to tweak the existing one. Fine tuning using organizational or purpose specific knowledge bases will again save on the huge costs involved in creating a new model.
Human Labour
Just as there are variation in the environmental impact of genAI applications based on their size and capabilities, there are also variations across frontier models in how they are trained. Some applications, like ChatGPT, have been trained using ‘reinforcement learning through human feedback.’ This kind of training involves humans reviewing a prompt and the generated output and using ranking or ‘up or down voting’ to give the model feedback about the accuracy and helpfulness of the generated output. In addition to training for the accuracy of outputs, workers are also used to review outputs against guardrails of appropriate content (‘content moderation’). While technology tools, including social media and generative AI, have long employed human workers for content moderation, OpenAI came under great criticism for outsourcing this practice to low-wage workers in Kenya. These workers sifted through toxic and explicit content with an aim of creating safer systems for the broader public without full consideration of their psychological wellbeing.
Another human labour issue that has raised concerns are the predicted job losses and job changes resulting from the adoption of genAI technologies in the workplace. Some professions welcome the technology as a significant contributor to improving skills and information processing, while others understandably worry about job losses and change (see CBC’s The National video panel discussion below).
Since we are relatively early in the adoption of these technologies within disciplines, the predictions for job losses, though numerous and wide ranging, are only speculations at best. Nevertheless, the numbers can give us a good idea as to how much of an impact we can expect to experience based on predictors. Take a look, for example, at the chart in Figure 2.3.1 from the World Economic Forum’s white paper Jobs of Tomorrow: Large Language Models and Jobs (2023) which predicts genAI’s impact on some jobs based on task analyses.
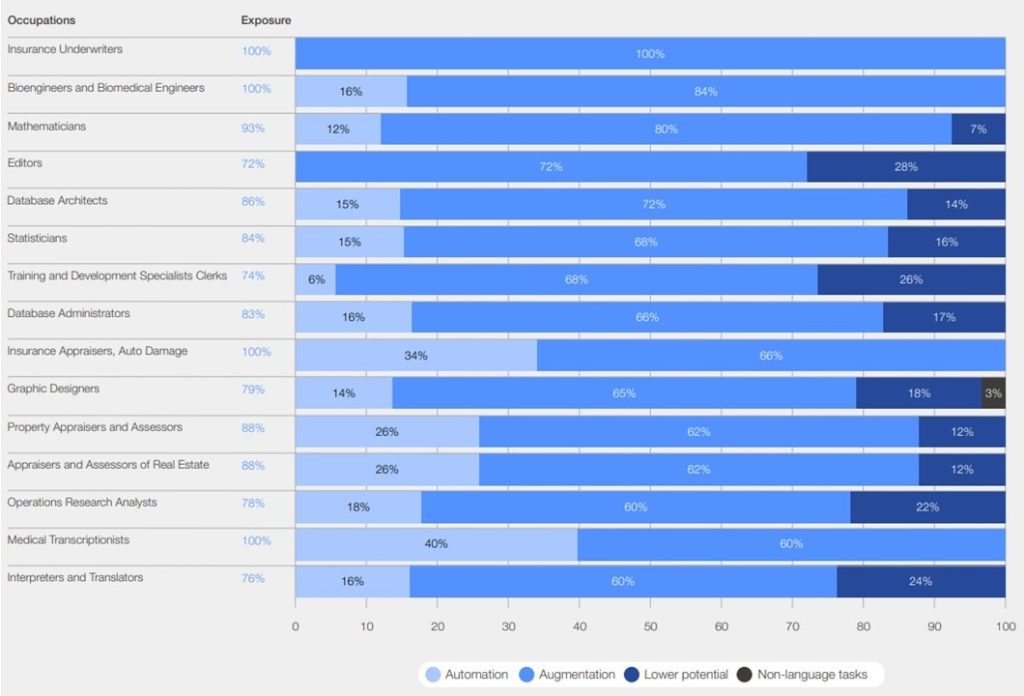
The many occupations listed will either be significantly affected by automation or will be augmented by genAI. Those occupations depicted here, however, represent a small slice of occupational sectors, and the data reported are to be taken as predictors only.
Take Action:
- Have conversations on the ethical development of genAI models and encourage your organization to work with genAI development companies that exercise ethical oversight in their upgrading process.
- Complete a deep dive into your career field to find out how your future job may be affected by genAI. Find out how the technology fits into day to day workflows. Does the type of work lend itself to genAI adaptation/augmentation or replacement? Perhaps you could learn how to use genAI to augment your knowledge and skills and to improve your employability.
Privacy and Safety
GenAI companies collect personal information from the time that a user visits their site until they leave it. At minimum, account data includes enough information to associate the individual with their account to login (this is usually name and email address). Sometimes setting up accounts includes providing additional demographic data that is either optional or mandatory. For services that require payment, the payment information directly associates the individual based on how they pay with the account and associated content making it harder to anonymize or alias the individual.
Types of Personal Data Collected by GenAI
Below is a listing of the types of personal data that genAI systems routinely collect.
Usage Data: The types of content requested, the types of content produced, features used, actions taken, time zone, country, dates and times of each request and response produced, user operating system version, user browser version, type of device used (computer, phone, tablet by brand and model), internet provider, IP address.
Device Data: As indicated above but without the details of how each feature was used with a device, but only the individual device information saved as a separate entry.
Session Data: Information about previous sites visited (i.e., cookies), the individual sites visited on the genAI company’s network, information about next sites visited, quality assurance data collected during site visits.
Log Data: Browser type, IP address, browser settings, date and time of using the service, how the user interacted with the functionality of the service.
All personal information ends up being associated with each individual’s account, and generated third party personal information is also associated with each individual’s account and linked to the use of services by that individual. The result is that an individual’s use of genAI services associates identifiable individuals with requests for products and may associate identifiable third parties within that identifiable user’s resulting products.
Watch this video to find out why privacy concerns are so important to every individual.
Privacy and safety issues extend to the use of the applications as well. Any organization making use of genAI technologies in Canada are guided by the Office of the Privacy Commissioner of Canada’s Principles for responsible, trustworthy and privacy-protective generative AI technologies. Organizations must also abide by the Freedom of Information and Protection of Privacy Act (FIPPA) requirements. These obligations also extend to employees. Though some genAI applications, such as ChatGPT, have settings that allow users to turn off data collection, which means the tool will not use the inputted prompts or data for later use or training, users still have an obligation to treat information they input in their prompts ethically and to proceed mindfully.
Take Action:
- Carefully review user agreements and understand the ways in which genAI applications may collect and make use of your data before consenting to use of the tools.
- Turn off the chat history and data training features, when the tool allows you to do so.
- Recognize that when engaging with genAI applications, you are responsible for the information you share with the tool.
- Do not share confidential or personal information or information for which you do not hold the copyright.
- Do not share colleagues’ work in part or in full using a genAI application without their informed consent.
- Do not share in part or in full information considered the property of the organization you work for (e.g., policies, internal documents, resources) unless there is a license or permission that explicitly allows such use.
- Review user agreements inclusive of privacy and data management policies because genAI companies are mainly private and assert ownership of data used in the services they provide.(Adapted from Centennial College, 2023).
Knowledge Check
Attributions
Content for this chapter has been partially adapted from the following sources. Considerable supplementary information has been provided by Robin L. Potter.
Centennial College. (2023). Privacy and data security. Centennial College – Generative Artificial Intelligence (GenAI) Guidelines for Faculty CC by 4.0
Center for Faculty Development and Teaching Innovation. (2023) What is Generative AI? Centennial College. What is GenAI? – Generative Artificial Intelligence in Teaching and Learning (pressbooks.pub) CC by 4.0.
Center for Teaching and Learning. (2023). Ethics, Data Privacy and Security, and FIPPA Considerations | CTL (durhamcollege.ca) CC by 4.0
KPU. (2023). Privacy in the Context of Teaching and Learning. Guidelines for Use – Generative AI (kpu.ca)
Paul R MacPherson Institute for Leadership, Innovation and Excellence in Teaching. (2023). General limitations and risks. Generative Artificial Intelligence in Teaching and Learning at McMaster University. McMaster University. General Limitations and Risks – Generative Artificial Intelligence in Teaching and Learning at McMaster University (pressbooks.pub) CC by 4.0
GenAI Use
Chapter review exercises were created with the assistance of CoPilot.
References
Aljezeera English. (2023, October). Who is the author of AI-generated art? | Digital Dilemma. Video. https://youtu.be/iPoRHiMLSOU?si=W-gogDb3dNm0f9oX
Carr, D. F. (2023, November 15). ChatGPT’s First Birthday is November 30: A Year in Review | Similarweb
CBC News. (2023, November). The Breakdown | Artificial intelligence is coming for your job. Video. https://youtu.be/3ipQ8XZb9ro?si=yB5L9-Z0TWxHMQhU
Cho, R. (2023). AI’s Growing Carbon Footprint – State of the Planet (columbia.edu)
CNBC International. (2023, December). A ‘thirsty’ AI boom could deepen Big Tech’s water crisis. Video. https://youtu.be/SGHk3zE5xh4DW Shift. (2023, July). https://youtu.be/zyhielIGpn4?si=b-XU2lV9ItbPnSBC
Crawford, K. (2024). Generative AI’s environmental costs are soaring — and mostly secret (nature.com)
DW Shift. (2023, July). AI spreading fake news. Video. https://youtu.be/zyhielIGpn4?si=_fNhjEnAC0P-GMUv
Government of Canada. (2022, updated). How to identify misinformation, disinformation, and malinformation (ITSAP.00.300) – Canadian Centre for Cyber Security
Hao, K. (2019). Training a single AI model can emit as much carbon as five cars in their lifetimes | MIT Technology Review
IBM Technology. (2023, June). Why large language models hallucinate. Video. https://youtu.be/cfqtFvWOfg0?si=uVAb5__cZ92MXLjj
KL3M. (2024). kl3m.ai – the cleanest LLM in the world
Kumar, A. and Davenport, T. (2023). How to Make Generative AI Greener (hbr.org)
London Interdisciplinary School. (2023, August 11). How AI image generators make bias worse. Video. https://youtu.be/L2sQRrf1Cd8?si=5rGRG5UCZaupFt_3
Ringman, M. (2023, September 6). Preventing Hallucinations In Generative Artificial Intelligence (forbes.com)
Shoaib, M. R., Wang, Z., Ahvanooey, M. T., and Zhao, J. (2023). Deepfakes, misinformation, and disinformation in the era of frontier AI, generative AI, and large AI models. IEEE International Conference on Computer Applications (ICCA), 2023. 2311.17394.pdf (arxiv.org)
Seneca Libraries. (2023). Artificial Intelligence – Copyright at Seneca – LibGuides at Seneca Libraries (senecapolytechnic.ca)
Smith, B. (2023). Microsoft announces new Copilot Copyright Commitment for customers – Microsoft On the Issues Blog.
Smith, C. (2023, September). What Large Models Cost You – There Is No Free AI Lunch (forbes.com)
The Associated Press. (2023, July 18). Margaret Atwood among thousands of authors demanding compensation from AI companies | CBC News
Wachter, S. (2019, May 6). Privacy, identity, and autonomy in the age of big data and AI – Sandra Wachter, University of Oxford. Video. O’Reilly. https://youtu.be/JvSEw1HuZvc?si=DGPiD9w8Lk3WHORZ
World Economic Forum. (2023). Jobs of tomorrow: Large language models and jobs. WEF_Jobs_of_Tomorrow_Generative_AI_2023.pdf (weforum.org)