2.6 Workflow and GenAI Co-Creation
Robin L. Potter
GenAI in Our Workaday Tasks
There is no doubt that genAI is having a significant impact on how we prepare our communications. Prior to large language models (LLMs) the typical workflow consisted of gathering information and resources, planning our documents, choosing software, collaborating, preparing the documents, obtaining feedback and revising accordingly, tracking versions, selecting the technology for distribution, and following up. With genAI in the mix, the process has either become more simplified or more complex, depending on your perspective and the AI tools applied to the tasks. Those with some familiarity and comfort with genAI use will see the applications as welcome assistants in the process of creating documents and completing related tasks as they can provide some ease in generating content and ideas, efficiency in drafting documents, images, charts, and tables, and in summarizing information, among other tasks. However, when considering the need for accuracy, detail, citations, and originality, the use of genAI may not offer as much of an increase in efficiency as one would like. So, understanding your workflow as you create communications on the job will help you to better integrate LLMs into the process. This chapter will define the concept of the workflow, discuss various degrees to which genAI and LLMs in particular can be integrated into workflows, and how to apply your evaluative judgement to maintain your agency through interactions.
What is a Workflow?
Simply put, a workflow consists of the sequence of steps involved in completing a task. Set within the communication context, such a workflow involves the process of preparing an intended communication, be it a message, document, presentation or other form of communication collateral. Whereas the writing process describes the cognitive sequence involved in creating the text or content itself (prewriting, outlining, drafting, revising, editing), the workflow usually encompasses the non-cognitive, more materials aspects. It is the act of drawing on resources at specific moments in the process such that they serve your individual purpose when needed the most. Interestingly, genAI fits within both the cognitive (e.g., ideation, content creation, coding) and the material (e.g., image and table generation, formatting, sequencing, genre conventions) processes involved in the creation of a communication.
Whereas for many decades workflow in business contexts referred to automated processes involving set procedures for the creation, review, and distribution of documents (Lockridge & Van Ittersun, 2020), the integration of genAI within business contexts is occurring on an individual employee basis with only 13% percent of companies surveyed by McKinsey in early 2024 making a concerted effort to implement genAI in a concerted manner (Relyea et al., 2024), indicating that employees are moving ahead with its adoption. In some cases, as we see in academic institutions, employees are adopting the technology within the constraints established by organizational policies and often through enterprise LLMs. Of note, however, is the secretive use of LLMs in workplace contexts where its use is undervalued or when excessive prohibitions have been established (Mollick, 2023). It is helpful, then, to look at genAI use within individual workflows, while understanding that each person would use genAI in different ways for various purposes. The concept of “workflow thinking” (Lockridge & Van Ittersun, 2020) becomes useful in this chapter as it allows us to step back and examine the role of not only LLMs but also of other AI technologies involved at various moments in the co-creation of communications. Applying workflow thinking, as opposed to simply mapping the process (which can vary greatly across individuals), will help us situate genAI in the co-creation process more broadly.
Integrating GenAI into Your Workflow
Workflows can be guided by departmental procedures and policies if the process is one that relies on collaboration with others. A workflow can be very personal, such as when you are working solo on an individual task, or it can bridge both the departmental and the personal as your work alternates between both solo and collaborative tasks. In an early 2024 survey, McKinsey researchers discovered a large percentage of respondents use internal or external generative AI in their daily work activities to help them with communication, creativity, critical thinking and decision making, as well as the ability to collaborate (see Figure 2.6.1):
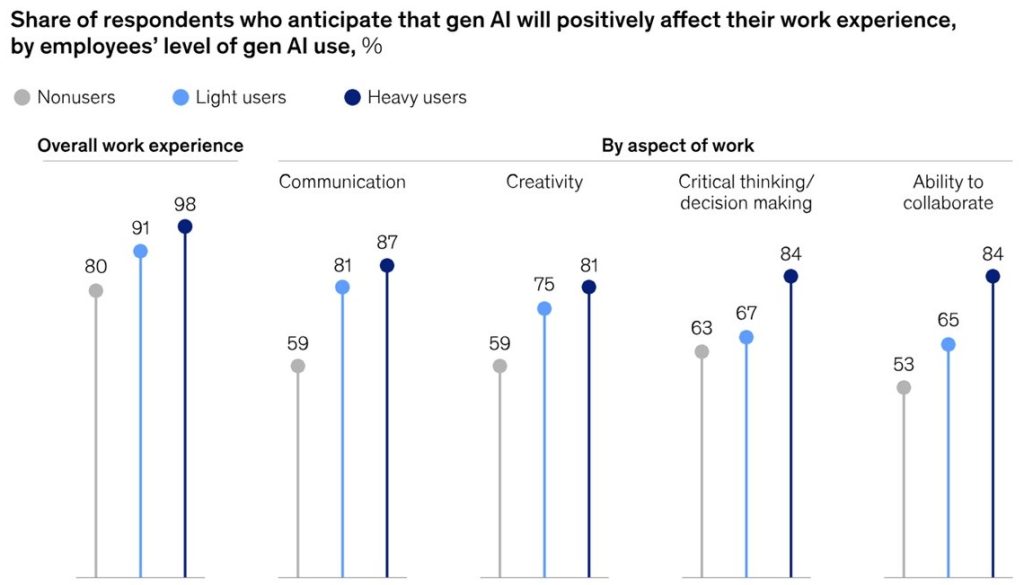
This graph depicts a broad range of genAI adoption in typical workday tasks to the benefit of employees, requiring some reflection on how, when, and for what and whom it is being applied.
Before integrating LLMs into your workflow, important factors, such as audience and purpose, environmental costs, potential for bias, and sentiment must be considered. Asking yourself these questions will help you decide whether to delegate the task to an LLM:
- Would engaging in the productive struggle (thinking through and revising the material) myself as I create the document reap more benefits than delegating the task to an LLM?
- What environmental or economic impact will my engagement with AI have, and is it worth it for the task at hand? (Keep in mind that using ChatGPT to draft one email message can cost the equivalent of one bottle of water [Crouse, 2024]).
- To what extent would I be using the content created by the LLM?
- What privacy and bias implications must I consider before using the LLM?
- How would my use of LLM content be influenced by copyright laws?
In his book, Co-Intelligence: Living and Working with AI (2024), Ethan Mollick suggests that we consider three approaches to using AI:
- Just Me Tasks: Some tasks are just best handled by humans. Although the various genAI models are getting better at doing so, often LLMs cannot convey sentiment in the same way that a human can, so when the message is of a personal nature, humans can do it best. Another reason for doing the writing yourself consists of the ongoing questions surrounding copyright relating to the LLM output. Until that is resolved by the courts, it’s best to ensure that the material you use is written using your own words. Finally, while some LLM versions are making inroads into reasoning through complicated problems, some tasks are so complex as to be best left to humans.
- Delegated Tasks: Some tasks may well be better delegated to an LLM. Asking it to draft an email message or a table, draft a report or essay outline, draft PowerPoint content, conceptualize a marketing campaign and the like would be typical effective uses of an LLM. For example, after you have done preliminary research, you can direct the LLM to generate ideas relating to a report topic and have them organized in an outline, then follow up with a second same request to obtain a different perspective allowing you to synthesize the options and come up with your own version. One caution is the question surrounding LLM output accuracy. Though the systems are improving over time, it’s always best to ensure that the content is accurate by corroborating any information you will use in your own work.
- Automated Tasks: A growing number of tasks can be automated by genAI, making mundane tasks less of a burden for humans. For example, you can ask an LLM like ChatGPT to generate survey questions along with the code that will automatically create the survey for you in a Google form (Levy, 2024) or you can ask it to summarize an article or analyze a large data set. It will also take data you input in the context window or upload in a file and create a chart that represents that data according to your criteria. Developments in the area of agentic AI are now also occurring. With agentic AI, AI agents or bots (specialized, task-focused software) will communicate with each other to solve problems and complete complex tasks. And the Claude model now has a beta version of the Computer Use agent that can take over a user’s computer upon direction to complete specified tasks. Release of such tools mark a whole new level of AI autonomy that will have significant impact on our society and the workplace.
Knowledge Check
Over time, genAI use in our day-to-day workflow will become more frequent and integral to the completion of both simple and complex tasks. The degree of separation between the human communicator and the LLM, however, will depend on how the LLM is integrated by each person into their workflow. And while this process will vary depending on the AI tool used, the degree of comfort in using the tool, the task and timeframe at hand, the subject matter, and organizational constraints (see Chapter 1.3 Understanding the Rhetorical Situation), one important consideration is how much agency or human control you are ready to hand over to the machine. Let’s consider this matter using Alan Knowles’ human-in-the-loop and machine-in-the-loop descriptions (2024). The following two use cases are good examples of how LLMs are being used within university contexts (but which could easily be applied to workplace contexts as well):
Damon’s Use Case (Human-in-the-Loop)
Damon begins his project in ChatGPT. He prompts the AI to give him a list of argumentative essay topics for a college writing course. He does not like any of the first 5 topics ChatGPT gives him, so he prompts it to generate a new list, from which he finds a suitable topic. Damon then prompts ChatGPT to generate an outline for an essay on the topic. After a few tries, he gets an outline he likes, complete with ChatGPT generated section headers. Next, he prompts ChatGPT to generate an essay based on the outline, then strategically works in some direct quotes from sources he located after choosing one of the generated outlines. As a final quality check, he proofreads the generated essay carefully and verifies as much of the factual information in the essay as he can before submitting it for a grade.
Knowles, 2024
In this example above, Damon has delegated much of his own research, writing, as well as creativity to ChatGPT. He is the human-in-the-loop, on the periphery of the co-creation process because he has the machine to do the following:
- Generate a topic list
- Create the essay outline and the essay
He asks the machine to do the bulk of the cognitive labour, meaning that he relinquishes his responsibility to engage in the process of human inquiry, resulting in a limited opportunity to exercise his own argumentative and analytical skills and creativity. He also makes use of a reverse citation method, which involves integrating quotes obtained from one of the outline versions generated by the machine, without doing the research himself, which should involve reading research papers, learning from the work done on the topic through these studies, and integrating quotes that represent arguments that align closely with the essay’s flow of ideas. Lastly, he does not verify all the factual information, which means that some claims in the version Damon submits may not be accurate. As you can see, there are so many gaps between the human and the machine in this workflow that it results in a final product that is questionable at best and scarcely represents Damon’s own thinking.
In the workplace, Damon’s process could jeopardize his own and the organization’s reputations by risking the release of information that may not be entirely accurate. In most contexts, this lapse may result in costly errors, and in contexts where safety is essential, the lapse may cause unintentional harm. Keeping the human at the forefront of the process is the most ethical way to proceed.
Let’s consider a different approach to a co-creation workflow:
Paige’s Use Case (Machine-in-the-Loop)
Paige begins her project on his [sic] university’s library webpage, where she performs keyword searches and uses Boolean operators to refine her searches. After skimming a few interesting articles, she settles on a topic. Next, she uses Illicit [sic], a tool that uses natural language input to find relevant peer-reviewed publications, for which it provides convenient one sentence summaries of those sources’ abstracts. Once she finds enough sources, she uses explainpaper, a tool that allows her to upload texts, then highlight portions of that text that will be summarized in the margins of the document. Next, she creates a detailed outline of his [sic] essay, including direct quotes and paraphrasing of all the sources included in her references section (some of these are edited versions of explainpaper summaries). She gives this outline to ChatGPT and prompts it to transform it into an argumentative essay, which she then proofreads and edits extensively before submitting.
Knowles, 2024
In an ideal world, this second example would be the workflow of choice for complex activities because in this process, while drawing on a variety of AI-enhanced software to assist, Paige remains the primary agent by doing the following:
- Using critical thinking skills to narrow the focus of the research and topic
- Integrating genAI applications at the very moment when they would be of best advantage: research, summarizing, drafting
- Including direct quotes and paraphrases drawn from her research
- Editing summaries and outlining
- Extensively editing and proofreading the draft content
Paige is the active human that employs AI software as tools; she does not delegate her power and control to the machine, as Damon appears to do. Through her process, she can legitimately claim ownership of the final product due to her efforts, especially since she has done the research, verified the output, and extensively edited the final text before submitting it. Paige appears to understand that there are just me tasks and makes use of the genAI applications for those tasks that would be best delegated for her purposes.
In the workplace, Paige’s process would keep the human in control of the information: from discovering the focus of inquiry to directing the LLM to perform specific tasks that are intended to assist rather than replace the employee. After carefully reviewing and extensively editing the output, the employee would have confidence in the accuracy of the content and of its alignment to organizational goals and values.
When comparing the two workflows, the human-in-the-loop use case involves a lesser or peripheral involvement of the human in the creation of content; whereas the machine-in-the-loop use case involves a greater degree of human agency or control in the creation of content, with the machine being more peripheral. In an ideal world, everyone one would keep the machine at the periphery of the work. The question you must ask yourself as you begin to interact with an LLM or any other genAI application is: “To what extent am I willing to hand over control over my workflow, my inquiry and ideation, and my content to the machine?” This will help you set your intention as you engage with the tools.
Knowledge Check
GenAI Co-creation
No matter what profession you work within, you will be affected by genAI technologies. Understanding the various ways in which these tools can be integrated into your workflow will lead to improved co-creation. Sun et al. (2024) studied genAI use in an organization involved in creative production. Though a small and limited study, it offers some insights into workflows that can be applied to other business types and industries. In particular, their Learning-Using-Assessing co-creative workflow framework is useful in describing how genAI is being integrated into individual workflows at this time when the technology is still being discovered by many.
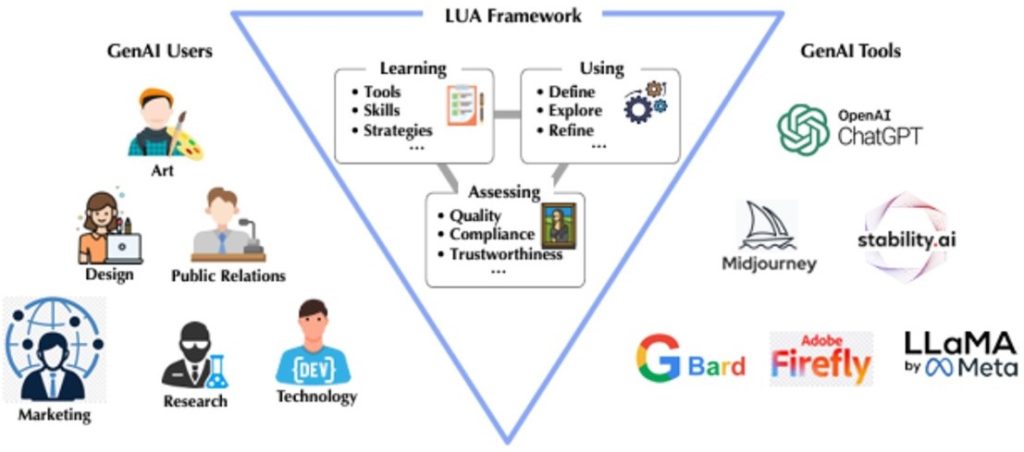
Sun et al. (2024) describe the human co-creation activity as comprising of several intertwined and sometimes concurrent activities involving the following:
Learning: In this activity, users are learning to use the genAI applications. This will involve learning new terminology and concepts, selecting and obtaining the necessary tools, and engaging in training or independent learning.
Many of us are very much in this phase of the process every time we engage with a new version of an LLM or a new model altogether. If you have never used Copilot, for example, you would want to learn about the various features, like voice (for mobile), file upload, and prompting. Learning about the application’s capabilities and features, how to prompt efficiently, the application’s limitations and quirks, and which genAI tools to use for specific purposes are all part of the process of gaining competence with this new technology. Finding reliable resources as well as coping with the ongoing updates to the model versions (and capabilities) are also challenges new users must overcome. Some strategies can include:
- Participating in professional training and completing learning modules on the use of LLMs and genAIs generally
- Accessing shared employee resources, such as prompt libraries, use cases and the like for reliable information on usage
- Consulting with your company’s AI champion, who can share tips on how to effectively make use of the technology for your role
Using: In this activity, users integrate genAI tools into their workflow. This process according to Sun et al. (2024) involves narrowing the focus of their interactions, engaging meaningfully with the genAI tools through iterative processes, and refining the responses and output.
When using Copilot, for example, we quickly realize that certain approaches, like refined and structured prompting yield richer results more quickly. You learn this by trial and error and intuition, and by applying the prompting principles covered in Chapter 2.4 and Chapter 2.5. Making use of human-AI collaboration verbs as shown in Figure 2.6.3 can also increase your interaction by requiring that the LLM keeps you in the centre of the co-creation process. Consider, for example, the difference between directing the LLM to “Solve X” compared to asking it to “Assist me in developing a solution for X.” In the latter case, you become an integral part of the problem-solving process making the engagement with the LLM substantially different than in the former case.
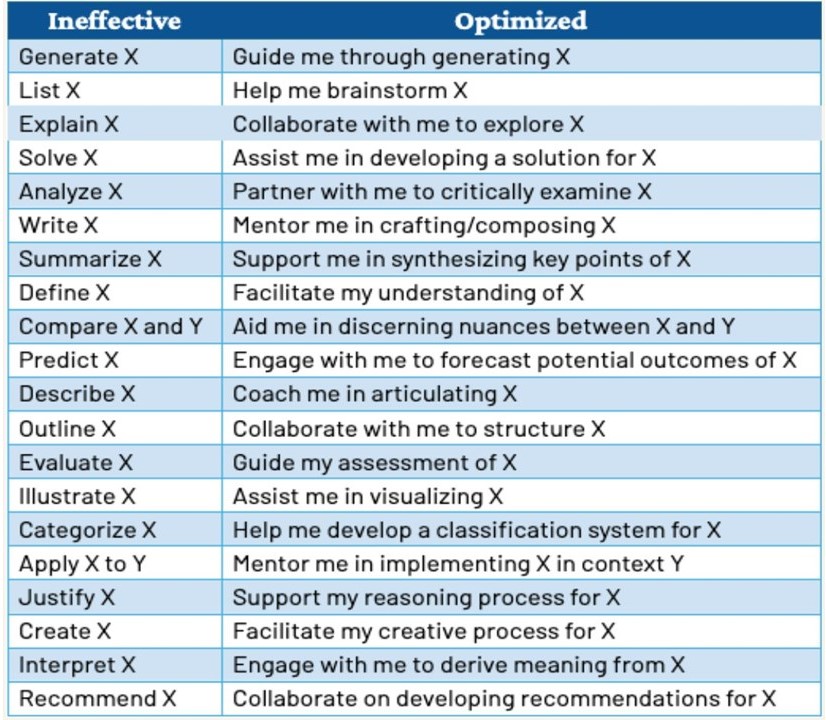
By reflecting on your workflow, you will be able to determine the best approach and moment to engage with the LLM and to which purpose. Being thoughtful in your approach and refusing to settle for only what is “good enough” will lead you to co-creating quality material with the LLM. You should be striving for excellence in all aspects of your work, especially when co-creating with an LLM. Engaging in an iterative process, using question and answer, and following up to refine the initial output will lead to better results—all around.
Assessing: Stepping back from the process, users can then assess both the tools and the outputs. Here, Sun et al. (2024) point out the necessity to evaluate usage in relation to quality, trustworthiness, and alignment with regulations and policies.
Every time you use Copilot, take a moment to reflect on your usage. Consider the following:
- How easy was it for you to obtain the output that served your purpose?
- How much effort in terms of iterations was required to achieve your goal?
- Was the output accurate, unbiased, inclusive, supported with verifiable citations?
- Would it have been easier for you to have done it yourself?
- Was this the right tool for the task?
If you repeatedly assess the process of working with the LLM, you will be able to adapt your own engagement and improve the process overall. Your assessment may result in a decision that use of the LLM for that particular task did not result in an optimal outcome. In that case, you will know that you either need to choose a different application or process or complete the task yourself. On the other hand, you may be entirely impressed by your co-created content. In that case, reflect on the strengths of the process and those actions that you want to repeat the next time you make use of the LLM.
Beyond Prompting: Claiming Your Agency in Co-creation
You might ask how you can maintain a sense of agency in the face of such a powerful technology. It is understandable to feel fearful that the machine will take over your job if you show the LLM how it is done, or that you have little control over the content that it can produce. It’s true that with each improved version of the LLMs, they have increased capabilities including those of persuasion and autonomy. And unless you have some knowledge of computer programming and can influence the development of the technology from the back end, as an end user you will feel that your power is limited.
Another concern with the increasing use of genAI and LLM applications is the negative impact that overuse can have on human creativity and the productive struggle. These technologies can both enhance and limit human creativity and when the work is handed over to the machine, humans are also relinquishing the important intellectual activity that is involved in the productive struggle. This struggle occurs when we engage in the cognitive work of sorting through a problem, revising our assumptions, taking an idea further, and learning new information in the process. This precious process is what lights up the mind. Being mindful of the important role humans have in the creation process can lead to more assertive engagement in the co-creation process. For these many reasons, intentionality and evaluative judgement are essential approaches to effective use of genAI applications.
Being intentional in your engagement with LLMs and other genAI applications involves bringing a sense of purpose that will focus the work. It also means that you bring to the interaction careful discernment that will guide you in applying your knowledge on how the application works, its weaknesses and strengths, along with the human practices and lapses that are all part of the process of learning to use the technologies.
Applying your critical judgement to both the engagement process and the review of output is also essential to ensure that you remain in the “driver’s seat.” Such an engagement can occur by employing what Tai et al. refer to as a sense of evaluative judgement, which “. . . is the capability to make decisions about the quality of work of self and others” (Tai et al., 2018). As they point out, exercising a sense of evaluative judgement involves having a sense of what constitutes quality in a work and being able to apply that determination to the work of self and others through the act of review. In their paper, Tai et al. go on to discuss how evaluative judgement is developed: through self-assessment, peer assessment and review, feedback, rubrics, and exemplars. These methods work well enough within an academic environment, but how do you engage in evaluative judgement in the workplace and in the face of genAI interactions? Some suggestions are offered below:
- Learning about standards of quality: Familiarize yourself with and work towards the standards of quality established by your department and/or organization so that the LLM output you use meets those standards. These standards of quality can be learned from current communications including current or archived documents, style guides, and policies (including genAI policies). Word of mouth from colleagues and established workflows are additional sources of information about quality.
- Routinizing revisions: Assume that all LLM output will require revisions: for accuracy, citations, and to make it your own. Build the revision process into your routine workflow so that it becomes a habit when receiving LLM output. Revising all LLM output allows you to not only make it your own, but also to ensure that the content you use from that output clearly aligns with the organizational values and goals. Review Chapter 2.5 for information on What to Watch For as you are revising the output.
- Applying your expertise, existing knowledge, and common sense: Make use of your own expertise, existing knowledge, and common sense to look critically at LLM output to determine its degree of accuracy and quality. You will be entering your employment with specialized knowledge that the organization is relying on you to apply to your daily tasks. Your expertise is the gateway to organizational and personal success. Apply your expertise gathered from your education and research, existing knowledge gathered from practices and experience, as well as common sense about what must hold to be true to make judgements about the quality of the work. Carefully scrutinizing LLM output for accuracy will lead to you make the necessary corrections so that your document reflects the standards of quality and accuracy that are to be met.
- Inviting peer review: Invite routine peer review of outgoing documents to ensure that all content—both human and co-created– aligns with the project and organizational goals and standards. Feedback from colleagues will help to ensure that hallucinations, bias, and privacy issues are mitigated before they do damage. Insights gained from the opinions of colleagues can help to deepen the development of content and ensure that the material is overall sound. Making use of feedback gained through the peer review process will lead to a stronger document overall.
Exercising your evaluative judgement at every moment of your engagement with LLMs and other genAI applications will help to ensure that you are at the centre of the co-creation process at both cognitive and instrumental levels in every phase of your workflow.
Determining how you will integrate genAI into your workflow, how you can engage with the technologies productively, and how to apply your evaluative judgement in the co-creation process will help you to produce content that will meet the standards of quality required to succeed in the workplace.
Knowledge Check
References
Crouse, M. (2024, September 20). Sending One Email With ChatGPT is the Equivalent of Consuming One Bottle of Water (techrepublic.com)
Knowles, A. M. (2024, March). Machine-in-the-loop-writing: Optimizing the rhetorical load. Computers and Composition. Vol. 71. Machine-in-the-loop writing: Optimizing the rhetorical load – ScienceDirect
Levy, D. (2024). ChatGPT Strategies You Can Implement Today | Harvard Business Publishing Education. Webinar.
Lockridge, T., and Van Ittersum, D. (2020). Writing Workflows: Beyond Word Processing. Writing Workflows | Title Page (digitalrhetoriccollaborative.org) and University of Michigan Fulcrum Press https://doi.org/10.3998/mpub.11657120. by NC-ND-4.0
Mollick, E. (2023). Detecting the Secret Cyborgs – by Ethan Mollick One Useful Thing Blog.
Mollick, E. (2024). Co-Intelligence: Living and working with AI. London, U.K.: Penguin Random House.
Pace Becker, K. (2024, August). Human-AI Collaboration Verbs. Table. Kimberly Pace Becker, Ph.D. on LinkedIn: If we want students to use AI for 𝗰𝗼𝗹𝗹𝗮𝗯𝗼𝗿𝗮𝘁𝗶𝗼𝗻 and… | 19 comments Also posted to Facebook in Higher Ed discussions of AI writing and uses, August 14, 2024.
Relyea, C., Maor, D., Durth, S., and Bouly, J. (2024). Gen AI’s next inflection point: From employee experimentation to organizational transformation | McKinsey
Ritala, P., Ruokonen, M., and Ramaul, L. (2024). Transforming boundaries: how does ChatGPT change knowledge work? | Emerald Insight Journal of Business Strategy.
Sun, Y., Jang, E., Ma, F., and Wang, T. (2024). Generative AI in the wild: Prospects, challenges, and strategies. Generative AI in the Wild: Prospects, Challenges, and Strategies (arxiv.org)
Tai, J., Ajjawi, R., Boud, D., Dawson, P., and Panadero, E. (2018). Developing evaluative judgement: enabling students to make decisions about the quality of work. Higher Education 76, 467–481. https://doi.org/10.1007/s10734-017-0220-3
GenAI Use
Chapter review exercises were created with the assistance of Copilot.